Do you feel like your selling job is becoming harder and harder over time?
Don’t worry — you are not alone: 69% of your colleagues feel the exact same way, according to Salesforce’s State of Sales 5th edition.
There are significant reasons why today’s fast-changing sales environment is making your job more difficult, including skyrocketing buyer expectations forcing sales reps to become trusted advisors, a switch from reactive adaptation to proactive prediction strategy, and widespread adoption of low-performing yet less risky sales techniques.
How to overcome these challenges, you ask?
Machine learning and artificial intelligence can handle them: analyze tons of data and make strategic predictions, cover multiple communication channels, provide valuable advice, and automate most of your routine tasks.
As the same State of Sales 5th edition claims, salespeople, on average, sell only 28% of their working hours.
So hurry up and learn how to stop wasting your time on inefficient routines and redirect your efforts to selling!
Key takeaways
- AI can enhance sales processes in many ways, from providing customer insights to automating tasks. However, human involvement is still critical for building relationships, understanding nuances, and ensuring ethical practices.
- Developing an AI strategy should involve identifying pain points, setting goals, assessing infrastructure, calculating ROI, researching solutions, piloting tools, training staff, and tracking performance.
- While AI provides many benefits like personalized recommendations and demand forecasting, it also poses challenges around data privacy, implementation barriers, reliability concerns, and job displacement fears.
- When evaluating AI tools, criteria like integration capabilities, user experience, output accuracy, transparency, data security, costs, and future evolution plans should be assessed against business needs.
- As AI evolves, sales processes will become more predictive and proactive. Virtual selling, role evolution, ethical practices, and immersive technologies will also emerge. However, human judgment and oversight will remain essential.
- AI adoption should focus on human-AI collaboration, not just automation. Sales professionals must direct AI tools, validate outputs, provide context, and maintain ethical standards.
- Sales enablement software remains vital for managing sales activities efficiently. AI tools complement such software through data-driven insights. However, core sales activities still require skilled sales professionals.
What is AI in sales?
Artificial intelligence (AI) in sales is about using machine-driven algorithms and processes to enhance and optimize sales operations.
At its core, AI analyzes vast amounts of data to identify patterns, make predictions, and offer recommendations.
Brief history and the evolution of AI in sales
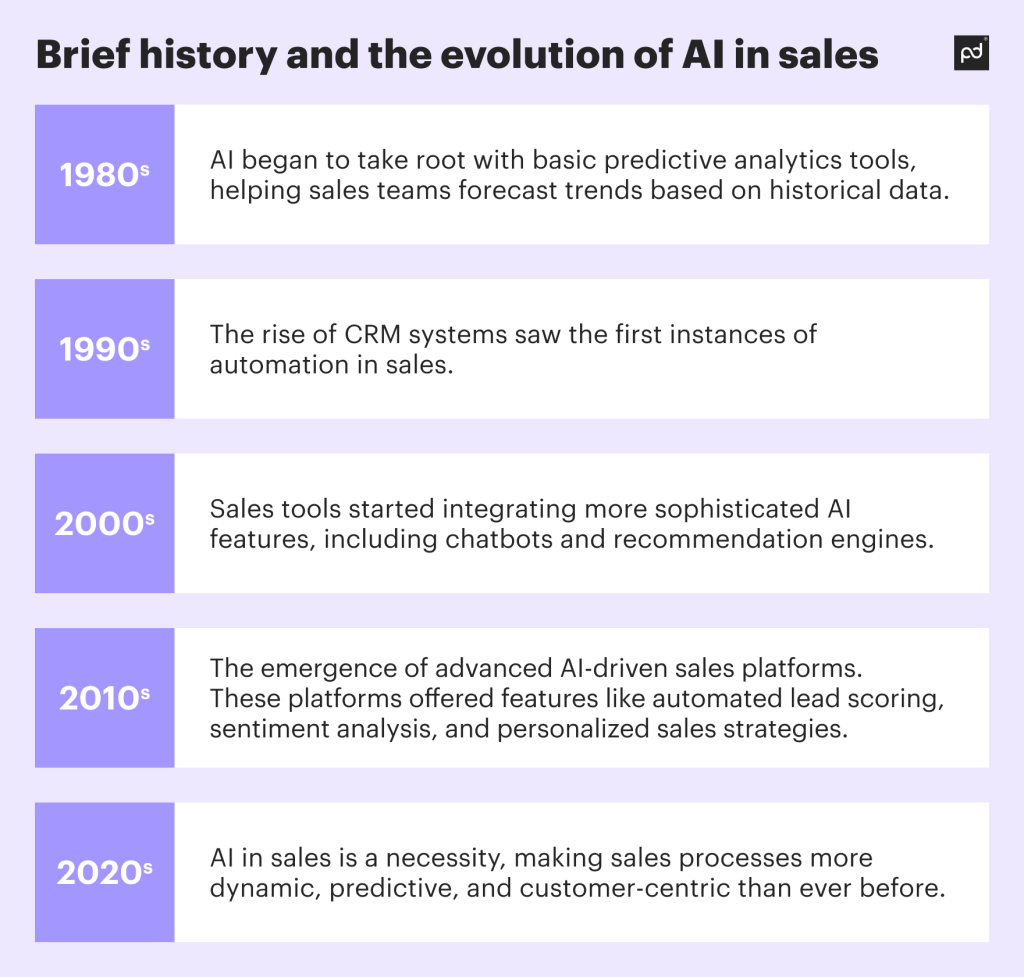
What are the benefits of using AI in sales
The integration of AI into sales processes offers a plethora of advantages, transforming traditional methods and driving efficiency.
Here’s a deep dive into the benefits, each with hypothetical examples.
Enhanced customer insights
AI analyzes vast amounts of data and can derive valuable insights about customer preferences, behaviors, and pain points.
Tech companies selling smart home devices can use AI to analyze customer reviews and feedback.
For example, an AI tool (like Brandwatch or Amplitude) identifies a trend: customers in colder regions appreciate the thermostats’ energy-saving features.
This insight allows the companies to tailor their marketing campaigns to highlight this feature in those specific regions.
As you can see, this deeper understanding allows you to craft strategies that result in higher sales numbers with less effort.
Improved sales forecasting
AI algorithms can improve sales forecasting and predict revenue streams with greater accuracy than people.
This is possible because AI examines much wider inputs like historical data, current market trends, and even external factors like economic indicators.
For example, fashion retailers can accurately predict the demand for winter coats.
By employing AI-powered analysis (like Salesken, Dealcode AI, or Freshsales), they can now immediately review past sales data, current fashion trends, and weather forecasts, which isn’t as feasible with just a manual approach.
Automation of routine tasks
You can trust a myriad of administrative tasks with AI, from data entry to scheduling follow-ups.
The automation potential goes far beyond what is typically considered possible.
It also opens a hidden benefit. With AI, sales teams can automate those important tasks for which they neglected to allocate resources due to their high labor intensity.
AI-powered CRM systems like Salesforce can automatically log details, adjust inventory, and set strategic follow-ups, which is simply impossible within a strict meeting schedule.
Better lead prioritization
AI-driven tools are much more advanced in scoring and ranking leads than typical sales representatives.
What’s important is that such tools can access and utilize parameters far beyond the usual set you’ve used to consider within the manual scoring.
Online B2B platforms employing sales enablement software can score leads not just based on interaction metrics like page views or downloads.
AI can also analyze patterns in content engagement, time spent on specific product pages, and cross-referencing data — company growth indicators and recent industry news mentions.
You can use specialized tools like Akkio Augmented Lead Scoring, or even more universal LLM chatbot-based tools like ChatGPT or Claude.ai.
Using chatbots and virtual assistants
Chatbots and virtual assistants powered by AI are excellent in initial customer interactions quite efficiently and with record-breaking average response time.
When visitors of travel agencies’ websites inquire about beach vacations, the chatbots provide instant package details, check availability, and book consultations with travel agents — all that the first-line support had done in the past.
For such purposes, you can use specialized or customized AI-powered chatbots like Chatbot or Wonderchat, as well as a more general GPT-3.5 Turbo with API and predefined inputs.
Agile pricing policy
AI can analyze market trends, competitor pricing, and demand fluctuations to suggest dynamic pricing strategies.
Dynamic real-time pricing is highly demanding yet heavily labor-intensive and risky in terms of setting the wrong price accidentally.
But AI can handle it with even a lower risk level than experienced sales reps can achieve.
E-commerce platforms selling thousands of items, for instance, simply can’t be supported by human-powered dynamic pricing.
Specialized AI-powered tools like Dynamic Pricing AI or Imprice, in turn, can monitor dozens of competitors and hundreds of thousands of parameters and react immediately.
Improved sales coaching and training
Rely on AI reviewing sales calls and interactions to identify areas of improvement and best practices.
It’s nearly impossible to review large batches of calls in a continuous manner manually.
But Colibri Sales Copilot and other similar AI software surely can.
Analyzing dozens of calls that an IT sales department makes daily, artificial intelligence identifies intricate trends — like newer reps that often struggle to explain particular features.
Sales managers then organize training sessions focused on these features, improving the teams’ pitches.
Personalization at scale
Manually crafting highly personalized messages for each customer segment at scale is almost always a huge challenge, as it requires tons of manual effort.
But with the power of AI, it’s much easier now to wrap your arms around this task.
Take a look at an example: Panda AI can instantly generate a personalized copy for each field of your sales proposal. All you need is to check and insert it.
The context for this copy can be borrowed from the block where the AI is added, your prompt, and the selected template.
For the cases that include crafting personalized messages, you can use ChatGPT or any other AI-powered conversational chatbots.
Developing an AI-powered sales strategy
To understand the transformative power of AI in sales, let’s cover how to develop an AI-powered sales strategy step-by-step.
We expanded each step with samples based on illustrative examples.
1. Identify current pain points in the sales process
This step helps you gain a clear understanding of areas that are draining time and resources unnecessarily.
For example, many sales teams spend several hours each day manually qualifying leads, which takes time away from engaging with high-value prospects.
Conducting an unbiased audit ensures you capture the true challenges faced across the sales team.
The goal is to document repetitive and time-consuming tasks that could be optimized or automated with AI.
- Start by conducting a thorough audit of your sales process.
- Run workshops or surveys to understand the daily challenges of your team members.
- Document repetitive or time-consuming tasks that could be streamlined with AI.
- Consider outsourcing this audit to a sales consulting company, a good option to get new expertise and overcome your possibly biased vision.
An AI-driven tool could:
- Automatically score and rank leads based on predefined criteria.
- Predict lead conversion probabilities based on historical data.
- Reduce the time sales reps spend on manual qualification, allowing them to focus on high-potential leads.
The company organized a series of focus group sessions. Each session included 8—10 sales reps from various regions and product lines. The sessions were facilitated by an external consultant to ensure unbiased feedback. They also used Wonderway.io AI-powered sales coaching software to leverage the sales process.
During these sessions, sales reps are asked about:
- Tasks that took up the most time in their daily routine.
- Parts of the sales process they found redundant or unnecessary.
- Challenges they faced in lead generation, qualification, and conversion.
- Tools or resources they felt would help them be more efficient.
- Sales reps expressed that:
- They spent an average of 3 hours daily qualifying leads.
- Almost 50% of the leads they engage with weren’t a good fit for the company’s products.
- Manual lead qualification often led to overlooking potential high-value clients.
They calculate the potential revenue loss:
- If a sales rep’s average hourly wage was $30 and they spent 3 hours daily on lead qualification, the direct cost was $90 daily per rep.
- With 50 sales reps, the total daily cost was $4,500.
- Annually, this amounted to $1,125,000 spent on lead qualification alone.
2. Set goals for AI adoption aligned with business objectives
Define specific, measurable outcomes you aim to achieve with AI.
Setting clear, measurable goals is crucial to drive focused AI adoption tied to business value.
The goals should be based on the pain points and opportunities identified in the sales process audit.
They must also align with overarching business objectives so that AI-driven improvements lead to tangible outcomes like increased revenue, lower costs, or improved customer retention.
For example, goals could be to reduce lead qualification time by 20%, increase sales team productivity by 15%, or boost customer lifetime value by 10% within a defined timeframe.
Having quantifiable metrics is key — rather than just aiming to “improve sales,” set targets like “reduce sales cycle by 5 days.”
An online retailer has noticed a plateau in sales despite increasing website traffic. They believed that enhancing the accuracy of their product recommendations could lead to higher conversion rates and, consequently, increased sales.
After analyzing its current product recommendation system, the company found that:
- The current accuracy of relevant product recommendations was at 60%.
- Only 1 in 5 visitors added recommended products to their cart.
- Feedback from customer surveys indicated a desire for more personalized shopping experiences.
The company’s broader business goal for the year was to increase online sales by 15%. Improving product recommendation accuracy is a key strategy to achieve this, as more accurate recommendations can lead to higher purchase rates.
Based on the initial assessment and alignment with the broader objective, the retailer set a specific goal: To improve product recommendation accuracy from 60% to 80% in the next quarter.
To understand the potential impact of this goal, they did some calculations:
- If 10,000 visitors are exposed to product recommendations daily, an improvement from 60% to 80% accuracy means 2,000 more visitors will receive relevant recommendations each day.
- Assuming a conversion rate of 5% for relevant recommendations, this could lead to 100 additional sales daily or 3,000 additional sales monthly.
3. Assess existing tech stack and infrastructure
Conducting a comprehensive audit of your current technology tools and platforms is crucial.
This is how you can clearly understand if the current infrastructure can support integration or requires an upgrade.
- List all sales and marketing software, CRMs, analytics tools, data storage systems, etc., in use.
- Identify limitations — does your CRM offer AI capabilities? Can data systems handle real-time analytics?
- Get input from IT, sales, and other teams on their existing pain points.
- Compare desired AI features against current tools’ abilities.
- Look at data security protocols as well — AI relies on quality, well-organized data.
For example, if you want AI for predictive lead scoring, assess if existing CRM can provide this or needs an upgrade.
Assessing infrastructure helps budget for upgrades like newer CRMs, cloud data storage, and real-time analytics APIs.
If expertise is lacking in-house, engage external consultants to audit systems.
A real estate firm has been keen on leveraging AI to optimize customer interactions and improve lead conversion rates. Before diving into AI adoption, they decided to assess their existing tech infrastructure.
The IT department created a comprehensive list of all software, platforms, and tools currently in use. This includes their CRM, website backend, customer communication tools, and data storage solutions.
The sales and marketing teams listed down the functionalities they expected from AI integration, such as predictive lead scoring, automated customer communication, and data analytics.
Comparing the current tech stack with the desired AI functionalities, the IT team identified that:
- The current CRM system could not integrate AI plugins.
- Their data storage solution wasn’t optimized for the real-time data processing required for some AI feature sets.
To validate their findings and get a broader perspective, the company hired an external IT consultancy firm that:
- Confirmed the limitations of the current CRM.
- Suggested potential CRM solutions in the market that are AI-compatible.
- Recommended upgrades to the data storage system to support real-time analytics.
Given the insights from the assessment and external consultation, the company’s management:
- Earmarked funds for upgrading to an AI-compatible CRM.
- Allocated budget for enhancing data storage and processing capabilities.
- Planned for potential training sessions for staff to familiarize them with the new systems.
4. Calculate ROI and budget for AI implementation
Conducting a detailed cost-benefit analysis is crucial in building the business case for AI investment and setting realistic expectations on ROI.
- Quantify all potential costs associated with AI adoption across software, hardware, integration, training, consultants, and staffing. Get help from finance teams or external experts to estimate expenses accurately.
- Then, estimate benefits through increased efficiency, cost reductions, revenue gains, and other metrics based on process improvements expected from AI.
- Compare costs versus benefits to determine ROI projections annually:
ROI = (net benefits / total costs) x 100
- Finally, decide between AI tools and set implementation budgets or timelines.
For example, AI could cut lead qualification costs by 20% through automation. It may take 12—18 months to break even, but project long-term gains.
A large restaurant chain was considering implementing an AI-driven inventory management system to optimize its supply chain and reduce food wastage. Below is a breakdown of how they calculated the ROI.
Annual food wastage costs: $500,000 (This includes spoiled ingredients, unsold dishes, and other food-related waste.)
Current inventory management system annual costs: $50,000
Total: $550,000
AI system setup and integration: $100,000 (one-time cost)
Annual AI system subscription: $70,000
Training for staff on the new system: $30,000 (one-time cost)
Total first-year costs with AI: $200,000
Total annual costs with AI (from second year): $70,000
By analyzing historical data and industry benchmarks, the company estimated that an AI-driven inventory management system could reduce food wastage costs by 15%.
Projected annual savings: 15% of $500,000 = $75,000
ROI calculation for the first year:
Total benefits (savings): $75,000
Total costs: $200,000
Net benefits (savings – costs): -$125,000
ROI = (-$125,000 / $200,000) x 100 = -62.5%
ROI calculation from the second year onwards:
Total benefits (savings): $75,000
Total costs: $70,000
Net benefits: $5,000
ROI = (net benefits / total Costs) x 100
ROI = ($5,000 / $70,000) x 100 = 7.14%
5. Research AI solutions tailored for key use cases and shortlist tools
Now, it’s time to research the AI tools market for efficient solutions covering your needs.
This in-depth research helps create a shortlist of solutions likely to provide the best ROI.
- Explore tools tailored to your industry and processes.
- Attend vendor demos to view options in action.
- Ask detailed questions to assess capabilities vs. needs.
- Read user reviews and case studies to learn real-world pros and cons.
- Reach out to companies using shortlisted tools for candid feedback.
- Evaluate ease of integration with existing tech stack based on audit findings.
- Compare costs like licensing fees, setup, and training. For instance, if automating lead qualification is a priority, research AI-powered lead-scoring tools.
- Assess features like lead rating, data analysis, and CRM integration.
- Shortlist tools that align with use case requirements have favorable reviews, and fit budget constraints.
A call center focusing on providing top-notch customer service recognized the potential of AI-driven voice analytics to enhance their operations. They embark on a journey to find the best tool for their needs.
A management team collaborated with floor supervisors to list down specific requirements:
- Real-time voice sentiment analysis.
- Call transcription with keyword highlighting.
- Post-call analytics for training purposes.
The team conducted an online search to identify leading AI-driven voice analytics tools. They created an initial list of ten potential solutions.
Over the next month, the team attended webinars hosted by these solution providers. They also request personalized demos to see each tool in action, focusing on their specific use cases.
The team also visited industry forums, read online reviews, and even reached out to other call centers using these tools. They gather feedback on:
- The accuracy and reliability of voice analytics.
- Ease of integration with existing call center software.
- Quality of customer support provided by the tool vendor.
For each tool, the team evaluated the cost of implementation and subscription against the potential benefits, such as reduced call handling time, improved customer satisfaction scores, and enhanced agent training.
Based on their extensive research, the company shortlisted three AI-driven voice analytics tools that best align with their needs and budget and received positive feedback from current users.
6. Conduct pilots of promising tools to test capabilities and ease of adoption
Before full-scale deployment, run controlled pilots using shortlisted AI tools with a small subset of users.
With preliminary real-world testing, you can validate the capabilities and ease of use of the tools which always look glossy yet do not always work as smoothly.
- Start with a limited rollout, like 20—50 users, for 1—2 months.
- Choose pilot participants across roles, regions, or product lines for diverse feedback.
- Then, develop quantifiable metrics to compare pre- and post-AI performance on factors like sales productivity, lead conversion rates, or call resolution time.
- Gather user feedback through surveys, focus groups, or interviews on satisfaction, challenges, and suggested improvements.
- Analyze real results vs. defined goals.This data-driven approach helps determine if the tool delivers the expected value. It also uncovers potential pain points in UX or integration to address before company-wide implementation, like specific blockers or complications in the workflows you have to automate or enhance with AI.
A finance company was eager to enhance its customer service and lead generation through an AI-driven chatbot.
Before fully integrating this technology into their website, they conducted a pilot test.
From their previous research, the company selected a chatbot tool that promises real-time customer query handling, lead capturing, and integration with their CRM.
The company decided to run the pilot for a month. They deployed the chatbot on two of their most visited web pages: “Home Loan Services” and “Credit Card Queries.”
A subset of the sales team, consisting of ten representatives, was trained on the chatbot’s backend. They learned how to monitor conversations, intervene when necessary, and extract lead information.
Over the month, the chatbot interacted with visitors, answering queries and capturing lead information. The sales reps monitored these interactions, occasionally stepping in for complex queries.
Two feedback forms were created:
- For customers — after each chatbot interaction, customers were prompted to rate their experience and provide comments.
- For sales reps — at the end of the pilot, the involved sales reps filled out a detailed feedback form about the chatbot’s accuracy, ease of use, and impact on their sales activities.
An analytics team assessed the chatbot’s performance by analyzing:
- The number of queries handled.
- Lead conversion rate through the chatbot compared to traditional methods.
- Average handling time for customer queries.
- Customer satisfaction scores from the feedback form.
The project team met with the sales reps to discuss their experiences. They delved into challenges, potential improvements, and the overall impact on their sales process.
Armed with quantitative data and qualitative feedback, the company’s management reviewed the pilot’s outcomes.
They assessed whether the chatbot met their expectations, its ROI potential, and any necessary modifications before a full-scale rollout.
7. Create training programs and change management plans for employees
Develop comprehensive training modules for each AI tool you plan to adopt.
Include both tool-specific and general modules.
- Tool-specific training covers functionality, workflows, data inputs etc.
- General training explains AI concepts, business applications, and benefits.
Training should enable employees to master AI for tasks like data analysis, decision-making, and customer service.
Hands-on practical sessions are ideal over just presentations. If expertise is lacking in-house, engage external AI trainers or tool vendors.
Training helps employees gain confidence in using AI-powered systems through practice in a safe environment.
It also highlights how AI helps them be more productive and develop new skills.
Beyond training, address change management concerns transparently via town halls and 1-on-1 meetings.
Provide reassurance on job security and continuous support during transition. The goal is to equip employees to adopt AI, not just operate tools.
A car dealership decided to leverage an AI tool to optimize its lead generation process. Recognizing the importance of proper training and change management, they implement a structured approach to ensure smooth adoption.
After selecting the AI tool, they organized an orientation session. The session aims to introduce the sales team to the tool, highlighting its benefits and the rationale behind its adoption.
The company partnered with the AI tool’s vendor to design a training program. The vendor provided insights into the tool’s capabilities, best practices, and common challenges users might face.
Based on the vendor’s input and the dealership’s specific needs, a series of training modules were developed, covering:
- Basic tool navigation and interface understanding.
- Advanced features tailored to lead generation.
- Troubleshooting and common issues resolution.
The sales team attended hands-on workshops to use the tool in a controlled environment.
These sessions, facilitated by the vendor and in-house experts, allowed the team to practice and ask questions in real-time.
The management team also identified team members who might be resistant to the new tool.
They organized one-on-one sessions to address concerns, emphasizing the tool’s benefits and the dealership’s commitment to supporting its staff during the transition.
8. Develop ongoing metrics and benchmarks to track the performance of AI
Establish a dashboard or reporting system to monitor AI’s impact continuously.
- Start with identifying KPIs that indicate progress towards your defined goals, like increased sales or higher productivity.
- Work with teams and tool vendors to build custom dashboards for real-time tracking.
- Integrate systems for making decisions, forming insights, or understanding a situation based on actual data.
- Review metrics to spot trends, anomalies, and opportunities for improvement. For instance, if you track lead conversion rates over time, you can optimize your AI lead-scoring models.
- Look at sales forecast accuracy and refine algorithms.
This performance tracking process keeps AI outcomes aligned with business needs rather than operating in a silo, allowing tweaking tools for better precision. It also provides ongoing proof of ROI to justify investments.
A leading fashion retailer integrated an AI-driven demand forecasting tool to optimize inventory management and reduce stockouts. Recognizing the importance of tracking the tool’s performance, they devise a structured approach to monitor its impact.
The management team, in collaboration with the inventory and sales departments, listed down critical KPIs to measure the tool’s effectiveness:
- Accuracy of demand forecasts.
- Reduction in stockout incidents.
- Inventory turnover rate.
- Cost savings from optimized inventory management.
The IT department collaborated with the AI tool vendor to develop a real-time dashboard. This dashboard visually represented the KPIs, allowing easy monitoring and quick insights.
To ensure the dashboard reflects accurate data, integrations were set up between the AI tool, the inventory management system, and the sales database.
- A recurring monthly review meeting was scheduled. In these sessions:
- The dashboard metrics were presented and analyzed.
- Any discrepancies or anomalies in the forecasts were discussed.
- Feedback from the sales and inventory teams was gathered to understand ground-level challenges.
- Strategies were devised to optimize the tool’s performance based on the insights.
Based on the monthly reviews, the management identified areas where the AI tool could be fine-tuned for better accuracy. They collaborated with the vendor for necessary updates or modifications.
Every quarter, the performance metrics were compared against the initial goals set during the AI tool’s adoption. This helped in understanding if the tool was on track to achieve the desired outcomes.
9. Continuously evaluate AI innovations and be ready to adapt as technology evolves
AI technology is evolving rapidly. To realize its full value, you must stay on top of the latest innovations and be ready to adapt to them quickly.
- Assign dedicated staff to track AI advancements through industry events, research publications, forums, etc.
- Visit industry conferences, subscribe to AI journals, and engage with AI communities. Be open to refining your strategy based on new insights.
- Attend demos and proactively reach out to vendors of promising solutions.
- Be ready to tweak strategies based on new solutions that align with goals. For example, if new NLP algorithms can improve automated lead classification accuracy, consider integrating them.
The aim is to build a sales tech stack that leverages cutting-edge AI advancements relevant to your needs. It is key to avoid stagnating with outdated tools when better solutions emerge.
A prominent logistics firm recognized the rapid pace at which AI technology is evolving. To ensure they remain at the forefront of innovation and harness the potential of new AI advancements, they implemented a proactive approach.
The company assigned a team member the specific role of AI research analyst. Their primary responsibility was to keep abreast of the latest AI developments relevant to the logistics industry.
Every month, the analyst attended at least two AI-related webinars. These webinars, often hosted by AI experts, tech companies, or industry associations, provided insights into the latest tools, techniques, and best practices.
Annually, the company sponsored their AI research analyst to attend major AI conferences. Here, they got the opportunity to witness live demonstrations, participate in workshops, and network with AI professionals.
The analyst subscribed to leading AI journals and magazines. These publications offered deep dives into recent AI research, case studies, and emerging trends.
The analyst became an active member of online AI forums and communities. These platforms allowed the analyst to engage in discussions, pose questions, and share the company’s experiences with AI implementation.
At the end of each month, the analyst organized a presentation for the team. They shared:
- Key takeaways from the webinars and conferences.
- Highlights from the journals.
- Discussions and insights from the AI communities.
Challenges and considerations of using AI in sales
While AI offers a multitude of benefits in the sales domain, it’s essential to recognize and address the challenges and considerations associated with its implementation:
Data privacy concerns
With AI systems collecting and analyzing vast amounts of customer data, there’s a heightened concern about data privacy.
Implementation barriers
Adopting AI in sales isn’t just about purchasing a tool. It involves organizational alignment, training, and overcoming technical obstacles.
Possible issues with reliability and accuracy
AI systems, while powerful, can make mistakes. It’s crucial to review their outputs and not rely solely on their recommendations.
Continuous learning and adaptation required
AI models thrive on continuous data inputs. They require regular tweaking and updating to remain effective and relevant.
Finding the right balance between automation and human interaction
While automation can enhance efficiency, it’s essential to maintain human touchpoints in the sales process.
Addressing fear of job loss
The integration of AI often raises concerns about job displacement within sales teams.
Cybersecurity risks
With AI systems processing vast amounts of data, there’s an increased risk of cyberattacks and data breaches.
Ethical considerations
Ensuring AI systems operate without bias and with transparency is crucial to maintaining trust and ethical standards.
Key criteria for evaluating AI tools
When considering the adoption of an AI tool for sales, it’s also crucial to have a clear set of criteria to ensure the tool aligns with your business needs and values.
Here’s a table-style guide to help you evaluate potential AI solutions.
Each criterion contains three possible results, where the first one is always the most desired, the second one is acceptable but not preferred, and the third one is unacceptable (though not necessarily for all cases).
Some of the criteria might not affect your context. You may exclude them from your evaluation.
Criteria | How to evaluate | Possible results |
---|---|---|
Integration with tech stack | Review the tool’s integration capabilities. Does it natively support your existing platforms? If customization is needed, consider the complexity and costs involved | — seamless integration — requires customization — incompatible |
User interface quality | Test the tool with a diverse group of users. Gather feedback on ease of navigation, clarity of features, and overall user experience | — highly intuitive — moderately intuitive — not user-friendly |
Accuracy of outputs | Run the tool through various scenarios and compare its predictions or outputs against known outcomes or benchmarks | — highly accurate — generally accurate — inconsistent accuracy |
Learning and improvement | Investigate the tool’s learning methods. Does it adapt based on new data? How frequently is the model updated? | — continuous learning — periodic updates — static model |
Training and support | Assess the vendor’s training materials, customer service responsiveness, and availability of resources to aid in tool adoption | — comprehensive support — limited support — no support |
Model transparency | Determine if you can access and understand the model’s decision-making process. Is the logic behind predictions clear and explainable? | — fully transparent — partially transparent — black box |
Ethical AI practices | Review the tool’s approach to avoiding biases, ensuring fairness, and other ethical considerations. Does the vendor have a clear stance on ethical AI? | — comprehensive ethical guidelines — basic guidelines — no guidelines |
Data security and privacy | Investigate the tool’s data handling practices, encryption standards, and access controls. Ensure compliance with relevant data protection regulations | — robust security measures — basic security — lacks adequate security |
Costs | Break down all potential costs, including initial setup, deployment, training, and ongoing fees. Compare against your budget and expected ROI | — one-time fee — subscription-based — variable costs |
Future evolution | Engage with the vendor to understand their vision for the tool. Are there plans for new features or improvements based on emerging AI trends? | — clear roadmap — vague plans — no future plans |
The future of AI-driven sales
As we look to the horizon, it’s evident that AI will surely redefine roles and introduce new paradigms. Here’s a glimpse into the potential future of AI-driven sales:
Predictive sales processes
With the continuous refinement of AI algorithms, sales processes will become increasingly predictive.
AI will analyze vast datasets to forecast customer needs even before they arise.
This proactive approach will enable businesses to offer solutions tailored to individual customer requirements, often before the customer has even identified a need.
Enhanced virtual selling
Virtual assistants, powered by AI, will play a more significant role in sales, handling everything from initial inquiries to closing deals.
These AI-driven entities will engage customers in natural language, providing information, answering queries, and negotiating terms.
Role evolution
With AI handling many of the routine and data-driven tasks, the role of sales professionals will evolve.
They will focus more on building deep customer relationships, understanding nuanced needs, and offering strategic solutions.
Ethical selling and transparency
With AI’s capability to analyze vast amounts of personal data, there will be an increased emphasis on ethical selling.
Companies must ensure they use data responsibly, maintaining transparency with customers about how their data is used.
Integration of augmented reality (AR) and virtual reality (VR)
AI, combined with AR and VR, will offer immersive sales experiences.
Customers can experience products or services in a virtual space, aiding their purchase decisions.
As you can see, the future of AI-driven sales promises a blend of technology and human interaction, where AI tools handle both data-driven decision-making and rote tasks and routines, and humans focus on supervising and correcting the AI efforts, as well as on enriching them with personal touch.
This synergy will drive sales to new heights, offering unparalleled customer experiences and business growth.
Wrapping up
AI tools can analyze vast amounts of data and make smart decisions, draw patterns, and make quite accurate predictions.
But the human element — understanding, empathy, and ethical judgment — remains irreplaceable.
As businesses integrate AI into their sales processes, they must ensure these tools are used responsibly.
Transparency in how customer data is used, ensuring fairness in AI-driven decisions, and avoiding biases are all highly important.
In essence, the future of AI-driven sales is bright and filled with opportunities and innovations.
But as we stride forward, let’s do so with a commitment to ethical adoption and a focus on the invaluable human-AI collaboration.
The power of AI itself isn’t a panacea for your sales.
Using robust sales enablement software to manage your sales activities is every bit as relevant and important today as it’s been in the past.
By adopting our solution, you can create, negotiate, and sign documents faster and with less effort.
Try it now by starting a 14-day free trial or requesting a free demo.
Disclaimer
PandDoc is not a law firm, or a substitute for an attorney or law firm. This page is not intended to and does not provide legal advice. Should you have legal questions on the validity of e-signatures or digital signatures and the enforceability thereof, please consult with an attorney or law firm. Use of PandaDocs services are governed by our Terms of Use and Privacy Policy.